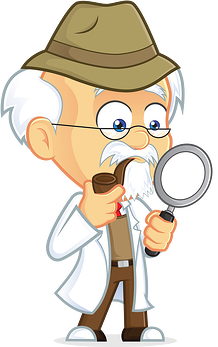
Dear Dr. Jay,
What’s hot in market research?
-Steve W., Chicago
Dear Steve,
We’re two months into my column, and you’ve already asked one of my least favorite questions. But, I will give you some credit—you’re not the only one asking such questions. In a recent discussion on LinkedIn, Ray Poynter asked folks to anticipate the key MR buzzwords for 2015. Top picks included “wearables” and “passive data.” While these are certainly topics worthy of conversation, I was surprised Predictive Analytics (and Big Data), didn’t get more hits from the MR community. My theory: even though the MR community has been modeling data for years, we often don’t have the luxury of getting all the data that might prove useful to the analysis. It’s often clients who are drowning in a sea of information—not researchers.
On another trending LinkedIn post, Edward Appleton asked whether “80% Insights Understanding” is increasingly "good enough.” Here’s another place where Predictive Analytics may provide answers. Simply put, Predictive Analytics lets us predict the future based on a set of known conditions. For example, if we were able to improve our order processing time from 48 hours to 24 hours, Predictive Analytics could tell us the impact that would have on our customer satisfaction ratings and repeat purchases. Another example using non-survey data is predicting concept success using GRP buying data.
What do you need to perform this task?
- We need a dependent variable we would like to predict. This could be loyalty, likelihood to recommend, likelihood to redeem an offer, etc.
- We need a set of variables that we believe influences this measure (independent variables). These might be factors that are controlled by the company, market factors, and other environmental conditions.
- Next, we need a data set that has all of this information. This could be data you already have in house, secondary data, data we help you collect, or some combination of these sources of data.
- Once we have an idea of the data we have and the data we need, the challenge becomes aggregating the information into a single database for analysis. One key challenge in integrating information across disparate sources of data is figuring out how to create unique rows of data for use in model building. We may need a database wizard to help merge multiple data sources that we deem useful to modeling. This is probably the step in the process that requires the most time and effort. For example, we might have 20 years’ worth of concept measures and the GRP buys for each product launched. We can’t assign the GRPs for each concept to each respondent in the concept test. If we did, there wouldn’t be much variation in the data for a model. The observation level becomes a concept. We then aggregate the individual level responses across each concept and then append the GRP data. Now the challenge becomes one of the number of observations in the data set we’re analyzing.
- Lastly, we need a smart analyst armed with the right statistical tools. Two tools we find useful for predictive analytics are Bayesian networks and TreeNet. Both tools are useful for different types of attributes. More often than not, we find the data sets comprised of scale data, ordinal data, and categorical data. It’s important to choose a tool that is capable of working with this type of information
The truth is, we’re always looking for the best (fastest, most accurate, useful, etc.) way to solve client challenges—whether they’re “new” or not.
Got a burning research question? You can send your questions to DearDrJay@cmbinfo.com or submit anonymously here.
Dr. Jay Weiner is CMB’s senior methodologist and VP of Advanced Analytics. Jay earned his Ph.D. in Marketing/Research from the University of Texas at Arlington and regularly publishes and presents on topics, including conjoint, choice, and pricing.