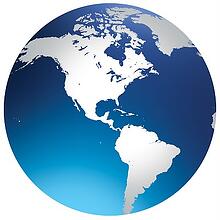
Guess what? It’s 2014! The year of Super Bowl XLVIII©, the 100th anniversary of the start of World War I, the 70th anniversary of D-Day, and a whole host of other, generally not-that-impactful events, anniversaries, and changes. One event that will happen in 2014, though, is something which happens every two years: U.S. national elections.This seems like an odd way to start a blog, but bear with me for a moment. Show of hands out there (ed. note: you’re welcome to actually raise your hand if you want, but I wouldn’t): how many of you readers have, at some point, become tired of the relentless political horse-race, always talking about who’s ahead and who’s behind for months and years on end? I know I have, and chances are it’s happened to you too, but I’m going to ask that we all take a deep breath and dive once more into the fray.
The question of “who’s ahead” and “who’s behind” brings us to our discussion of statistical significance. I’m going to talk today about how it works, how it can be used, and why it might not be quite as beneficial as you might think.
First, a quick refresher: when we take survey responses, test results, etc. from a sample of people that we think represents some broader population, there is always the risk that whatever results we see might be due to random chance instead of some other factor (like actual differences of opinion between two groups). To control for this, we can conduct significance testing, which tells us the likelihood that the result we have obtained is due to random chance, instead of some other real, underlying factor. I won’t bore you with the details of terms like p, α, one- vs. two-tailed tests and the like, but know that the methodology is sound and can be looked up in any AP-level statistics textbook.
Most organizations assume an “error range” of 5%, meaning that a data finding is statistically significant if the odds are 5% (or less) that the results are due to random chance. So, if we run significance testing on Millennials vs. Gen X’ers in a survey, and we find that the two are significantly different, we are saying there is a 5% (or less) chance that those differences are just random, and not due to actual underlying opinions, or price-sensitivity, or political beliefs, or receptiveness to that new hair-growth prescription, or whatever else you might be testing.
Now, if you have a huge data set and a fairly advanced statistical program, calculating significance is easy. But since most people don’t have access to these tools, there is another, much simpler way to think about significance: the margin of error. The margin of error is a simple way of determining how much higher or lower a result can be before it is considered significantly different. For instance, if your margin of error was ± 5%, and your data points were 60% and 49%, your data is (likely) significantly different; if your data points are 55% and 51%, they are not.
This brings us back to the political analogy; calculating the margin of error is how we determine whether Politician X is ahead of Politician Y, or vice-versa.
Let’s say, for example, a poll of 1,000 registered voters was conducted, with a sound methodology, and asks which of two candidates respondents support (assume no other options are presented in this circumstance, a small but notable difference for a future blog). We find that 48% support Politician X and 52% Politician Y. Because the sample size is 1,000, the margin of error is ± 3.1%. Since the difference between the two politicians is less than twice the margin of error (i.e., if Politician X’s share might be as high as 51.1% and Politician Y’s share as low as 48.9%), you would hear this reported as a “statistical tie” in the news. This would be because news organizations won’t report one candidate as ahead of the other, as long as the two are within that acceptable margin of error.
So that’s the political world, and there are many reasons networks and polling organizations choose to behave this way (aversion to being wrong, fear of being seen as taking sides, and fear of phone calls from angry academics, among others). But in the research world, we don’t usually have nice, round sample sizes and two-person comparisons – and that’s why relying on statistical significance and margin of error when making decisions can be dangerous.
Let’s go back to that political poll. The original sample size was N=1,000 and produced a margin of error of ± 3.1%. Let’s see what happens when we start changing the sample size:
· N=100: ± 9.8%
· N=200: ± 6.9%
· N=500: ± 4.4%
· N=750: ± 3.6%
· N=1,000: ± 3.1%
· N=1,500: ± 2.5%
· N=2,000: ± 2.2%
· N=4,000: ± 1.6%
Notice the clear downward trend: as sample sizes grow, margins of error shrink, but with diminishing returns.
Now, we at CMB would advocate for larger sample sizes, since they allow more freedom within the data (looking at multiple audiences, generally smaller error ranges, etc.). It’s no secret that larger sample sizes are better. But I’ve had a few experiences recently that led me to want to reinforce a broader point: just because a difference is significant doesn’t make it meaningful, and vice versa.
With a sample size of N=5,000, a difference of 3% between Millennials and Gen X’ers would be significant, but is a 3% difference ever really meaningful in survey research? From my perspective, the answer is a resounding no. But if your sample size is N=150, a difference of 8% wouldn’t be significant…but eight percentage points is a fairly substantial difference. Sure, it’s possible that your sample is slightly skewed, and with more data that difference would shrink. But it’s more likely that this difference is meaningful, and by looking at only statistical significance, we would miss it. And that’s the mistake every researcher needs to avoid.
If I can leave you with one abiding maxim from today, it’s this: assuming some minimum sample size (75, 100, whatever makes you comfortable), big differences usually are meaningful, small differences usually are not. Significance is a nice way to be certain in your results, but we as researchers need to support business decisions with meaningful findings, not (just) significant ones.
Nick Pangallo is a Project Manager in CMB’s Financial Services, Healthcare, and Insurance practice. He has a meaningful-but-not-significant man-crush on Nate Silver.