Dear Dr. Jay,
How can I explain the value of data integration to my CMO and other non-research folks?
- Jeff B.
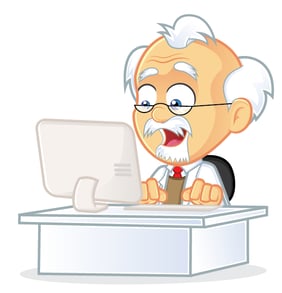
Hi Jeff,
Years ago, at a former employer that will remain unnamed, we used to entertain ourselves by playing Buzzword Bingo in meetings. We’d create Bingo cards with 30 or so words that management like to use (“actionable,” for instance). You’d be surprised how fast you could fill a card. If you have attended a conference in the past few years, you know we as market researchers have plenty of new words to play with. Think: big data, integrated data, passive data collection, etc. What do all these new buzzwords really mean to the research community? It boils down to this: we potentially have more data to analyze, and the data might come from multiple sources.
If you only collect primary survey data, then you typically only worry about sample reliability, measurement error, construct validity, and non-response bias. However, with multiple sources of data, we need to worry about all of that plus level of aggregation, impact of missing data, and the accuracy of the data. When we typically get a database of information to append to survey data, we often don’t question the contents of that file. . . but maybe we should.
A client recently sent me a file with more than 100,000 records (ding ding, “big data”). Included in the file were survey data from a number of ad hoc studies conducted over the past two years as well as customer behavioral data (ding ding, “passive data”). And, it was all in one file (ding ding, “integrated data”). BINGO!
I was excited to get this file for a couple of reasons. One, I love to play with really big data sets, and two, I was able to start playing right away. Most of the time, clients send me a bunch of files, and I have to do the integration/merging myself. Because this file was already integrated, I didn’t need to worry about having unique and matching record identifiers in each file.
Why would a client have already integrated these data? Well, if you can add variables to your database and append attitudinal measures, you can improve the value of the modeling you can do. For example, let’s say that I have a Dunkin’ Donuts (DD) rewards card, and every weekday, I stop by a DD close to my office and pick up a large coffee and an apple fritter. I’ve been doing this for quite some time, so the database modelers feel fairly confident that they can compute my lifetime value from this pattern of transactions. However, if the coffee was cold, the fritter was stale, and the server was rude during my most recent transaction, I might decide that McDonald’s coffee is a suitable substitute and stop visiting my local DD store in favor of McDonald’s. How many days without a transaction will it take the DD algorithm to decide that my lifetime value is now $0.00? If we had the ability to append customer experience survey data to the transaction database, maybe the model could be improved to more quickly adapt. Maybe even after 5 days without a purchase, it might send a coupon in an attempt to lure me back, but I digress.
Earlier, I suggested that maybe we should question the contents of the database. When the client sent me the file of 100,000 records, I’m pretty sure that was most (if not all) of the records that had both survey and behavioral measures. Considering the client has millions of account holders, that’s actually a sparse amount of data. Here’s another thing to consider: how well do the two data sources line up in time? Even if 100% of my customer records included overall satisfaction with my company, these data may not be as useful as you might think. For example, overall satisfaction in 2010 and behavior in 2015 may not produce a good model. What if some of the behavioral measures were missing values? If a customer recently signed up for an account, then his/her 90-day behavioral data elements won’t get populated for some time. This means that I would need to either remove these respondents from my file or build unique models for new customers.
The good news is that there is almost always some value to be gained in doing these sorts of analysis. As long as we’re cognizant of the quality of our data, we should be safe in applying the insights.
Got a burning market research question?
Dr. Jay Weiner is CMB’s senior methodologist and VP of Advanced Analytics. Jay earned his Ph.D. in Marketing/Research from the University of Texas at Arlington and regularly publishes and presents on topics, including conjoint, choice, and pricing.