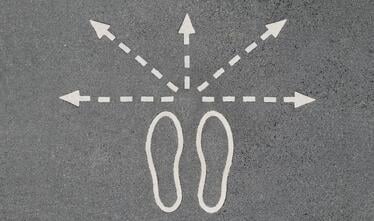
One chilly night in February, I was heading home from a friend’s birthday festivities when my car just stopped working. I had just enough oomph and momentum from the hill I was on to pull off to the side of the road. I found myself stranded in the middle of the city, waiting for a tow truck until 4AM and vowing to myself the whole time that I wouldn’t deal with this clunker anymore. It was time for a new car. During the next two weeks, without wheels, I did my research on the Internet and made my way over to a local Toyota dealership. I walked in knowing exactly what I wanted: a 2014 green Corolla. I even knew the various payment and financing options I was prepared for. And wouldn’t you know it—I ended up getting exactly what I said I wanted.As easy as that sounds, my path wasn’t straight to the doors of the Toyota dealership. I had gone through a variety of different makes, models, financing options, and colors. At the end of researching each car, I asked myself not only if I would really buy this car, but also if I would truly be happy with it. It wasn’t until I asked myself this question for the first time that I realized I was essentially creating my own Discrete Choice Measurement (DCM), specifically a Dual-Choice DCM (DCDC).
DCM is a technique that presents several configurations of product features to respondents and asks them to pick which configuration they would most prefer. In a Dual-Choice DCM, a follow-up question is asked to determine whether the respondent would actually buy the preferred package. This second question is crucial—I might choose a Lamborghini but there’s little chance (OK, no chance) that I will actually purchase one.
Dual-Choice DCM scenarios are the gold standard for product development work and can lend more accurate insights into a buying scenario by:
- more closely representing a consumer’s purchase decision
- helping us better understand consumer preferences
- more accurately reflecting market potential
- dissecting the product into pieces, which allows us to measure price sensitivity and willingness to pay for the product as a whole as well as individual components
- simulating the market interest in thousands of potential product packages for product optimization as the analysis examines how a product can be changed to perform better by identifying (and tweaking) individual product features that affect purchase decisions
Being able to produce more realistic results is obviously an important part of any research, and it just goes to show that DCMs can truly help with any decision making process. Running a DCM in my head prior to purchasing my car was truly helpful, so it’s no surprise that our clients often rave about the DCMs and Dual-Choice DCMs in our analytics program.
Heidi is an Associate Researcher who graduated from Quinnipiac University with a dual-degree in Marketing and Over-Involvement. After realizing she lacks hobbies now that student organizations don’t rule her free time, Heidi is taking sailing classes and looks forward to smooth sailing on the Charles River by the end of the summer.
Want to know more about our advanced analytic techniques, including our innovative Tri-Choice Approach? Let us know and we’ll be happy to talk through how we choose the right techniques to uncover critical consumer insights. Contact us.